By Sophie Lange, News Editor
On Wednesday, April 10, the annual Hanson Lecture titled “Creative Solutions to Persistent Problems: Confronting Race, Gender and Ability Bias in Tech” was held in the CUB Ballroom. Associate Provost for Faculty Affairs Michelle Schmidt welcomed the audience and explained that the Hanson fund supports a lecture each year featuring a prominent national or international figure with the intention of furthering Gettysburg College’s educational goals. Associate Professor Dr. Meredith Broussard of the Arthur L. Carter Journalism Institute at New York University was the selected lecturer for this year.
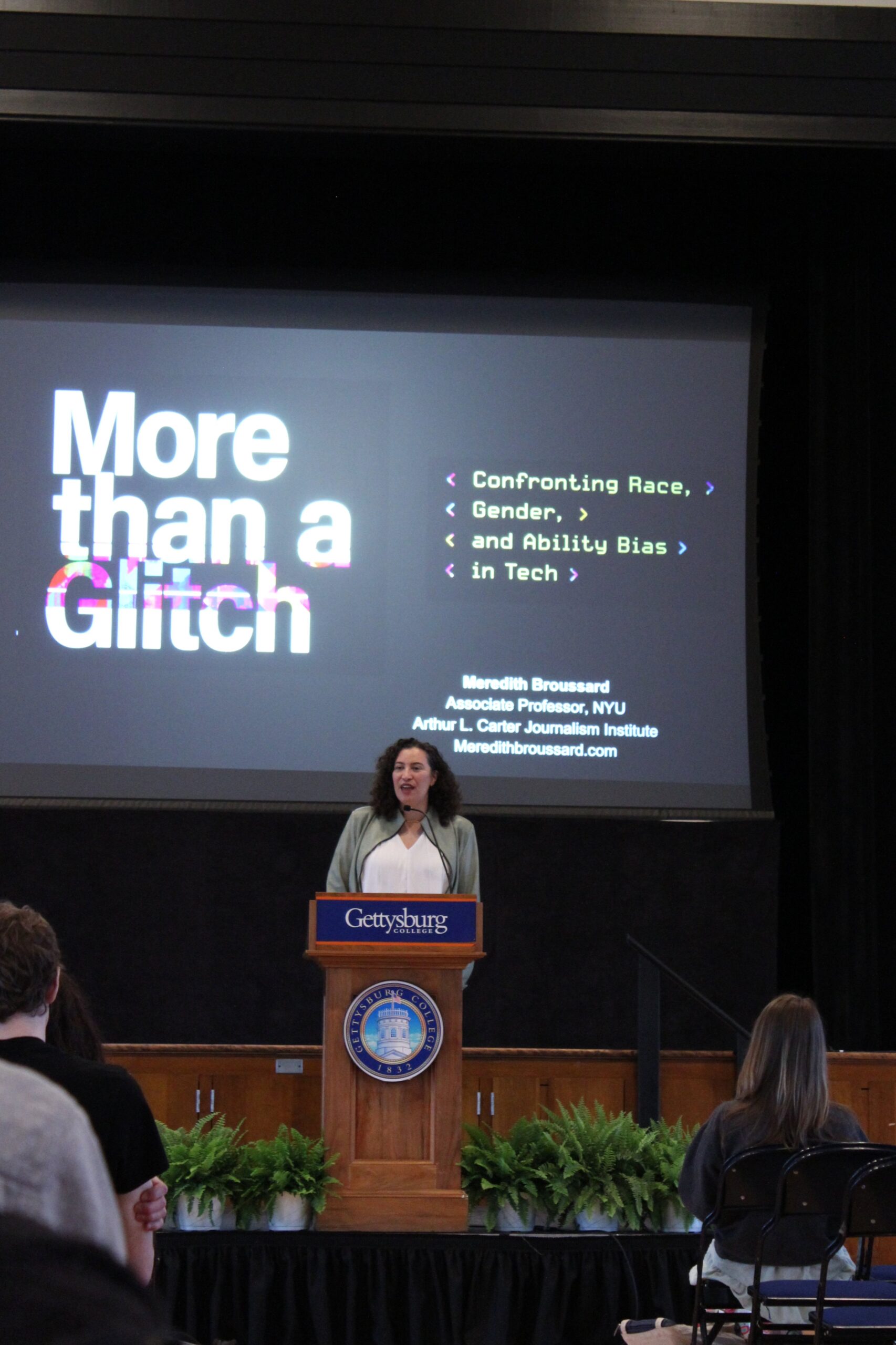
Associate Professor Dr. Meredith Broussard of the Arthur L. Carter Journalism Institute at New York University was the selected lecturer for this year. (Photo Grace Jurchak/The Gettysburgian)
Broussard began her lecture with a discussion about Hollywood’s depiction of artificial intelligence (AI) and how it is drastically different from what AI actually is: math.
“It’s really important to recognize that Hollywood images are not real AI. They’re imaginary. What’s real about AI is that AI is math. It’s very complicated, beautiful math,” Broussard said. “So if any of you were worried about a robot takeover, I hope this is a little reassuring because math is really great, but it’s not going to rise up and take over anytime soon.”
She continued to discuss the fact that mathematical definitions are often not the same as societal definitions. She used the example of a cookie that needed to be split between two children to describe fairness. In this situation, a computer would define fairness as each child receiving 50% of the cookie. However, in reality, the cookie would likely be split into one bigger half and one smaller half.
“When I was a kid, my brother and I would fight about who got which half of the cookie, so if I wanted the big half of the cookie I would say to my brother, ‘All right, you let me have the big half of the cookie now; I will let you pick the TV show that we watch after dinner.’ And my brother would think for a second he would say, ‘Yeah, that sounds fair.’ And that was a socially fair decision,” Broussard explained.
She said that while computers can calculate the mathematically fair choice, this is not the same as social justice. Broussard said this “explains why we run into so many problems when we try and calculate solutions to really long standing social problems.”
AI is a type of machine learning, Broussard explained. For machine learning to be successful, large amounts of data must be uploaded onto a computer. Using the patterns found within this data, the computer is able to make a model that has the ability to perform a wide range of tasks, such as making predictions or decisions. In addition, AI can use this data to generate images, audio and text, hence the term “generative AI.” She briefly discussed how AI uses predictions to execute the aforementioned tasks.
Broussard described the ways in which AI technology uses datasets known as “common crawl.”
“Common crawl was assembled by using what are called spiders, or web crawlers, and the way that this works was you started what parlor on one web page is a one web page has a bunch of links on it,” Broussard said. “The crawler grabs a copy of that webpage and sticks it into its pocket into the database. And then it goes to every single link on that page and grabs a copy of the page there and then goes to every single link on every single one of those pages and grabs a copy and sticks it in the pocket, and you do this for long enough and you have millions, billions of web pages.”
She explained that while the common crawl data is referred to as being “clean,” the technology definition is different from the societal definition.
“Now we’re into the distinction between what the social definition of clean is and the data science definition of clean because the social definition of clean to me says, ‘Alright, this data has been curated. It has been purged of problematic things… and the data science definition of clean data is tidy,” Broussard clarified.
Because this uncurated training data is used to perform tasks, structural discrimination is built into generative AI in what is now known as “techno-chauvinism.”
“The problem is when social problems get embedded in an AI system, they become very difficult to see and even more difficult to eradicate,” Broussard said.
She illustrated several examples of discrimination in AI. The first was the use of AI technology in automated mortgage approval systems. Because mortgages have been disproportionately denied to borrowers of color throughout history, these automated systems are 40% to 80% more likely to deny loans to people of color than their white counterparts according to a recent study.
“What is the big sin historically that we know about in the American Housing Market? Redlining, restricting access to who could buy houses in particular neighborhoods. We also see in the footprint of American neighborhoods a very long history of residential segregation. So what these algorithms are doing is they are replicating this historical bias,” Broussard explained.
Next, she discussed bias in facial recognition technology, a topic studied by the Gender Shades Project. The research found that the technology was better at identifying individuals with light skin than those with dark skin as well as better at recognizing men than women. Transgender and nonbinary individuals were often not recognized at all.
“We could make visual recognition more accurate by including a greater range of skin types and genders in the training data, [and] yes, it would make it more accurate, but we don’t necessarily want to do that. Because let’s think about the uses of facial recognition. There are high-risk and low-risk uses of facial recognition,” Broussard added.
She gave examples of how this could affect individuals who were not as easily recognized, particularly with the use of facial recognition technology in policing.
“What’s going to happen then? Well, it’s going to misidentify women and people of color more often. They’re going to get caught up in the justice system unnecessarily, and we know that technologies such as facial recognition are disproportionately weaponized against communities of color and poor communities, against marginalized communities. And so, a more just solution or socially just solution is probably not to use facial recognition and policing,” Broussard expressed.
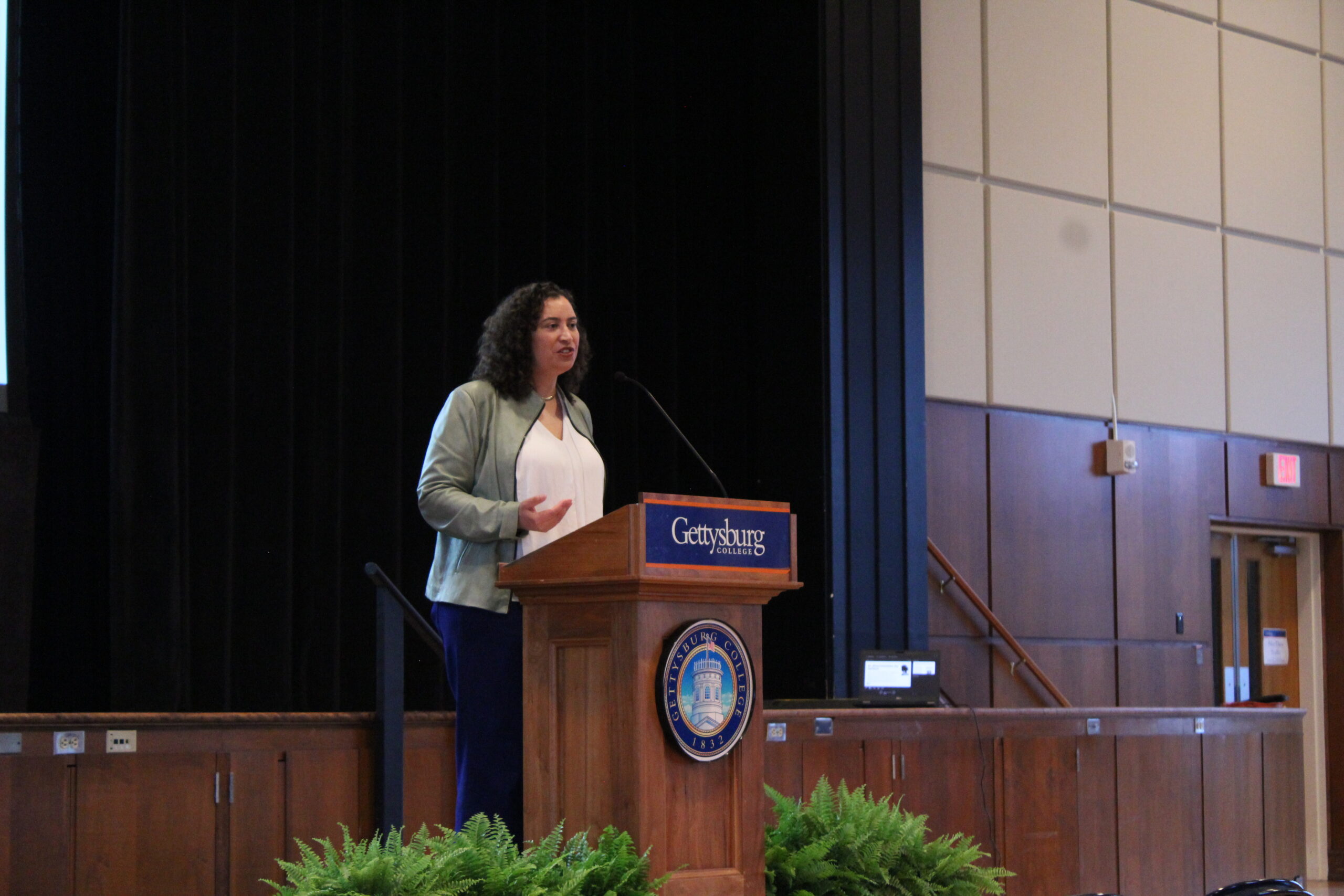
The annual Hanson Lecture was titled “Creative Solutions to Persistent Problems: Confronting Race, Gender and Ability Bias in Tech.” (Photo Grace Jurchak/The Gettysburgian)
Broussard’s next example involved the use of AI to sort through job applications for Amazon. In looking at the resumes of employees who were successful at Amazon, the system inadvertently began rejecting job applications of women, graduates of women’s colleges and female athletes.
On this topic, Broussard said, “Silicon Valley has a long-standing, well-known diversity problem… The model was just reflecting this pre-existing bias, and something that is important to note is that it’s often unconscious. Right? So I don’t think that the developers of this system started out saying, ‘I want to make something that’s going to impress people.’ I don’t think they started saying, ‘I want to kick out all the women.’”
Finally, Broussard explained the ways in which AI technology has discriminated against individuals with disabilities. She began by discussing how curb cuts not only help those with disabilities but also those without disabilities, such as people with strollers, and in recent years, delivery robots. In the example she gave, Broussard described a story in which a delivery robot threatened someone’s life. This particular robot was programmed to wait in the curb cut until the light turned green. She explained that while able-bodied people move out of the way, when someone with a wheelchair needs to get up onto the sidewalk, the robot was not programmed to handle that.
“The person who was trying to cross the street in the wheelchair was up against traffic, oncoming traffic, [and] was left stranded in the street, which is an incredibly dangerous situation, and I don’t think it’s one that the developers were trying to create. I think that the developers have just had unconscious bias. They were not thinking about designing for people in wheelchairs, they were thinking about designing for the able bodied majority,” Broussard said.
Finally, she expressed the issues with AI and misinformation, particularly about the upcoming election. Broussard discussed a recent study that evaluated the inaccuracy, bias, harm and completeness of the election information that generative AI provided across all major platforms. It found that 51% of the AI responses were inaccurate in terms of information about voting in the upcoming election in November.
Broussard gave the audience several pieces of advice regarding the development of AI technology.
Broussard’s first piece of advice was that “we can emphasize AI reality instead of dwelling in the realm of imaginary AI… Those things distract from the actual harms being experienced by actual people at the hands of AI today.”
Next, Broussard explained that “we can look for human problems inside AI systems. Instead of assuming that an AI system is not going to discriminate, we can assume that an AI system is going to discriminate. We can look for that problem in an AI system, and as a reporter who works on algorithmic accountability issues, I can assure you that it is like shooting fish in a barrel… Those problems are there to be found.”
She also added that collaboration is key to finding and solving these issues and recommended that individuals pay close attention to journalism and policy surrounding algorithmic accountability.
She concluded her lecture by providing the audience with a list of books and documentaries about AI technology that she recommends before giving a brief Q&A session with those in attendance.